Let’s continue our conversations around ML/AI in Service Assurance. I want to to explore an illustrated real life use case. The first example focus in on is around fault storms management. When bad things happen, they may create an explosion of faults. Each fault may be a separate situation. This operational overload is best described by a customer of mine — “a see of red”.
Impact of Fault Storms on Operations

When fault storms occur they cause many operational problems. First they cause blindness. It makes pre-existing problems and follow-on problems to get mixed in. Suddenly you have a mess. It may take hours to sort out responsibility alarms with manual correlation. Next they cause automation gridlock. Most service impacting alarms are set to immediately generate tickets. If 1,000 alarms try to open tickets at the same time, you may break your ticketing solution. Last they cause mistakes. Due to the human nature of sorting out the problem, errors are common. Operations can ignore a separate problem by assuming its part of another root cause. Fault storms, while rare, are dangerous for operations in assuring customer services.
Addressing Fault Storms with Machine Learning and AI
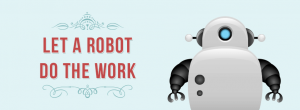
Fault storms are a great use case for ML/AI technology. Machine learning sets the bar for a “storm”. Artificial intelligence can create the situation by encapsulating all the service impacting faults. This isolation/segment would mitigate the “sea of red”. When storms occur, the solution mitigates the blindness. The storm situation is isolated from pre-existing faults and all follow-on problems. Automation would only run on the situation created by ML/AI. This avoids the overload scenario. Fault storms are rare, but can devastate NOC performance. ML/AI technologies are a great choice to mitigate them.
Mitigating Effects Fault Storms
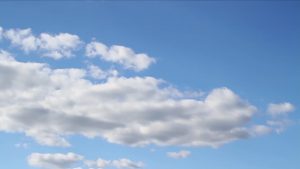
The best way to illustrate how this technologies works is by showing a solution to a problem. For example, a site outage. We you have a power outage at a remote site, its devastating. All services depending upon infrastructure are no longer available. There are hundreds fo service impacting alarms. The final result is a complete mess for operations to clean up. Now ML/AI can address the fault storm caused by the site isolation. All the alarms could have the same location field key, then have a commonality. The count of alarms from that location is tracked. Machine learning can built a model based upon those records. The rush of faults breaks that model. Then the result is an anomaly centered upon that specific location. The anomaly encapsulates the situation – all the service impact alarms. With a processed alarm list, the “sea of red” becomes “clear as a bell”. Operations can assign the single site isolation to an individual. Then after validation, the user can perform action — dispatch. Instead of confusion and panic, operations continues to move forward like any other day. Business as usual, no stress should be the goal.
Take Aways on Fault Storms
Fault storms can break people’s day. They invite failure by operations. At the grand stacks of hundreds of outages to spotlight will be overwhelming. Operations has the opportunity – will the die or will they shine. Leveraging ML/AI technology can keep them on rails. Then success will be the standard operating procedure.
Article Map
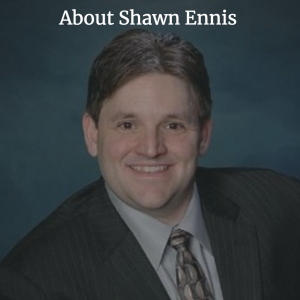
About the Author
Serial entrepreneur and operations subject matter expert who likes to help customers and partners achieve solutions that solve critical problems. Experience in traditional telecom, ITIL enterprise, global manage service providers, and datacenter hosting providers. Expertise in optical DWDM, MPLS networks, MEF Ethernet, COTS applications, custom applications, SDDC virtualized, and SDN/NFV virtualized infrastructure. Based out of Dallas, Texas US area and currently working for one of his founded companies – Monolith Software.