Well thats a wrap for my initial journey into machine learning and artificial intelligence. But it’s only the end of the beginning for machine learning. Before I finish up this series some people have asked that I provide some context. This technology is changing the industry. Some players have already adopted these technologies – my company and competitors. So to placate the masses, let’s list out what I have heard is currently available in the marketplace. I am not omnipotent, so if you see anything missing or wrong please let me know.
Artificial Intelligence is Old Hat
Let’s first talk legacy. Artificial intelligence has been part of software since my start of the industry. The most common of which are “rules”. Humans define the model though. This model could be a list of “if” statements. A tree stored in a database is also a model. The difference is not on the AI side, but the machine learning. Automated model building is what is different. Other legacy concepts are algorithm based. Two examples I have for you: linear regression trending and Holt-Winters smoothing. Both are available in open-source like MRTG as well as many commercial applications today. The commonality is that the algorithm provides the model. Let’s be clear, the algorithms doesn’t build the model, it IS the model. These are robust and well regarded solutions in the marketplace today.
Anomaly Detection vs Chronic Detection
Now lets move to machine learning. Anomaly detection, with various degrees of accuracy, is getting to be common in the marketplace. Many are black boxes that strain credibility and others are open time abyss of customization. The mature solutions are trying provide a balance between out-of-the-box value and flexibility. There are plenty of options with anomaly detection. Chronic detection and mitigation is much more rarer. I have not seen many who offer that functionality, especially accomplished with machine learning. Again on dealing with chronics, you mileage may vary but its out there.
Takeaways
Many of the products that use this technology do not specifically reference it. Usually when you hear analytics nowadays you can expect machine learning to be part of it. Most performance alerting (threshold crossing) leverage it in the realm of big data analytics. Most historical performance tools leverage machine learning to reduce the footprint of reporting. These three areas commonly have machine learning technology baked in.
What this means is that machine learning is NOT revolution technology that solves all our problems. At least not yet. Its revolution technology that lowers the bar. Because of this technology problems can be solved easier with far less resources than ever before. The price you pay for this is simple, machine learning will not catch everything. You will have to be fine with a 80% quality with 0% effort.
Thanks again for all the great input, keep commenting and I will keep posting.
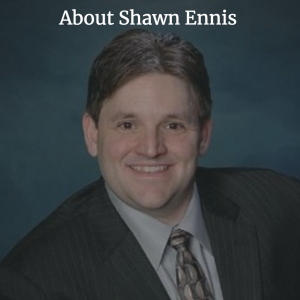
About the Author
Serial entrepreneur and operations subject matter expert who likes to help customers and partners achieve solutions that solve critical problems. Experience in traditional telecom, ITIL enterprise, global manage service providers, and datacenter hosting providers. Expertise in optical DWDM, MPLS networks, MEF Ethernet, COTS applications, custom applications, SDDC virtualized, and SDN/NFV virtualized infrastructure. Based out of Dallas, Texas US area and currently working for one of his founded companies – Monolith Software.