High ROI IoT and Edge Solutions for Sustainability
Earlier this year, a Gartner® report mentioned: “Previously, most enterprises viewed sustainability as a reputational overlay, which affected mostly marketing and communications. Nowadays, a growing number of companies see sustainability as a strategic parameter that directly affects how they do business and run their operations.”[1] Why? Because it’s a money maker. A well-crafted corporate environmental sustainability practice increases sales, investment, brand equity, and profitability.
Here are some of the ways that the IoT and Edge team at Concentrix Catalyst helps companies meet their sustainability and financial goals:
- Agriculture: We provide smart agriculture and precision farming solutions to reduce soil erosion and degradation, as well as reduce water and food waste
- Hospitality: We help restaurant chains reduce food, energy, and equipment waste by monitoring and optimizing kitchen staff protocols
- Real estate: We can monitor and reduce energy consumption in commercial buildings, rented or owned
We find that many are surprised to learn that edge solutions for sustainability are also affordable and easy to implement. Here’s additional detail on each:
Agriculture
In a traditional farm setting, the farmer needs to physically be in the field each day to monitor the equipment, assess its condition, and take readings. With equipment manufactured by a variety of vendors, farmers must learn to use multiple software tools to configure each piece of equipment. In addition, there is an industry-wide underutilization of scientific data for effective farming; for example, many irrigation systems continue to sprinkle water, even when it’s raining. An increasing number of farmers are turning to precision control and monitoring capabilities that allow them to manage their business more efficiently—freeing up time and money for other undertakings in an industry with notoriously small profit margins. Concentrix Catalyst developed central mobile-enabled application able to monitor, control, and manage operations across the farm, freeing the farmers from the need to physically assess conditions and control equipment on their farms. The solution enables farmers to monitor soil moisture and manage ancillary tools, sensors, and devices, regardless of manufacturer. A farmer can access the system, which helps utilize critical resources effectively by calculating scientific data, via desktop or a mobile app.
Streaming audio and video with a command-based interface allows her to view field conditions in real-time and respond accordingly.
Hospitality
A well-known worldwide fast-food franchise needed to reduce waste. Kitchen equipment was prematurely ending up in landfills due to accidental, but irreparable, damage by untrained operators. Food, like fryer oil, was being wasted due to the inefficiency of kitchen technology and employee practices. The restaurant chain hired Concentrix Catalyst to analyze kitchen operations using edge and Internet of Things (IoT) technology. We used edge and IoT to collect and analyze data on kitchen equipment and kitchen staff protocols, resulting in optimization recommendations for over 45,000 restaurants globally.
Google Coral is a leader in edge computing solutions like this, as Coral devices are affordable and the platform processes data very quickly.
Real Estate
Heating, ventilation, and air-conditioning (HVAC) systems in medium and large industrial and office buildings ensure safe, healthy, and comfortable conditions for occupants. A large office park or university campus can consist of hundreds, or thousands, of HVAC units. Industry estimates purport that these systems account for 35 percent of and 40 percent of commercial building energy consumption worldwide. Accordingly, maximizing the efficiency of these systems can result in considerable savings for an organization.
To leverage IoT and edge solutions for sustainability, Concentrix helped a specialty manufacturer of industrial application control valves design precision control of commercial heating and air conditioning systems. They wanted to help organizations better manage costs related to commercial heating and air conditioning control systems. Statistical analysis of data collected via climate-control systems and existing building automation systems affords the opportunity to control the climate of a building or group of buildings more precisely. It also reduces the resources needed for troubleshooting and addressing problems with the HVAC system due to central control of the systems and the ability to better pinpoint issues with the system.
We helped create a streamlined data pipeline that allowed the client to collect the information required, display the correct information to the clients who owned the data, and give engineers access to devices from a central location. A web app allowed for access to the precision-control system, and Catalyst designed the embedded system’s software for control and optimization of sensors and control valves—the software layer, or interface, with which the client’s customers and technicians interacted.
The end solution included an extensive feature set that allowed multiple consumers to benefit. C-level executives could view information about the building at the campus or building level to ascertain where money was being spent on energy use. Because the solution worked with existing building-operation systems, building operators could log into the app to better control building climates, choosing when to override the existing automated controls and responding to occupant comfort. A field engineer suite allowed technicians to monitor, analyze, and update the control system, and enabled remote management and firmware updates for the system hardware—reducing the time and resources needed to send engineers out to examine individual connections for problems.
Good for business. Good for the planet.
Sustainability and profitably are not mutually exclusive, and both are necessary to compete in the modern market. Companies that carefully plan their sustainability strategy and leverage technologies, like IoT and Edge, realize meaningful improvements in sustainability while also improving margins.
For more information about edge solutions for sustainability, and how our Sustainable Experience Engineering (SEE) practice can help your organization with its corporate environmental sustainability efforts, please contact us.
[1] Gartner, Competitive Landscape: Sustainability Consulting,., January 18, 2022
GARTNER is a registered trademark and service mark of Gartner, Inc. and/or its affiliates in the U.S. and internationally and is used herein with permission. All rights reserved.
Applying Web3 Concepts
Web3, the buzzy term for the next internet era based on blockchain technology, can foster a better customer experience in many use cases. For enterprises, a turnkey hosted decentralized application framework with the trust and privacy of blockchain built-in can enable better B2C applications, since the very design of blockchain allows companies to be everywhere all the time. When done right, Web3 concepts can be used to create personalized and frictionless experiences for consumers, while still guaranteeing privacy and security.
Below, we’ll break down a few examples of practical uses for Web3 concepts.
Web3 concepts in action
Let’s say you want to create a certificate of authenticity for a physical product you market, manufacture, and sell (like Nike does), or It could be a “badge” indicating attendance at an event (looking at you, AMC). To tie customers to your brand, you need a record of ownership that customers find value in now and over time. In a Web3 world, that’s called an NFT—non-fungible token. The art and card trading worlds are currently reinventing themselves with this technology, but it can also be used by enterprises.
This digital artifact can be given to a customer to keep in their digital wallet (that is, their blockchain user account). When they want to resell that product or prove ownership, it’s available 24/7/365 at no cost to you (the manufacturer) or them (the owner).
What do you need?
The math in a real-life example can be useful. Let’s look at what you need for execution:
- Omnichannel registration portal
- Marketing data reporting solution, including revenue
- Track and trace engine, to zoom in/zoom out of the data
This would cost you around $250k a year in a hosted software as a service (SaaS)-type framework. But what would that buy you? Say you want five limited edition runs monthly of around 25k NFT “badges.” The quantity changes barely affect the cost, but the results would be 1.5M NFTs a year, handed out to your customers digitally. You can charge for these items as you would for certificates of authenticity to the end customer or embed it in the cost. You can also add a “tail” to the NFT, in that when a transfer happens, you get a portion of the third-party sale as compensation for its creation. This feature could enable the solution to effectively pay for itself.
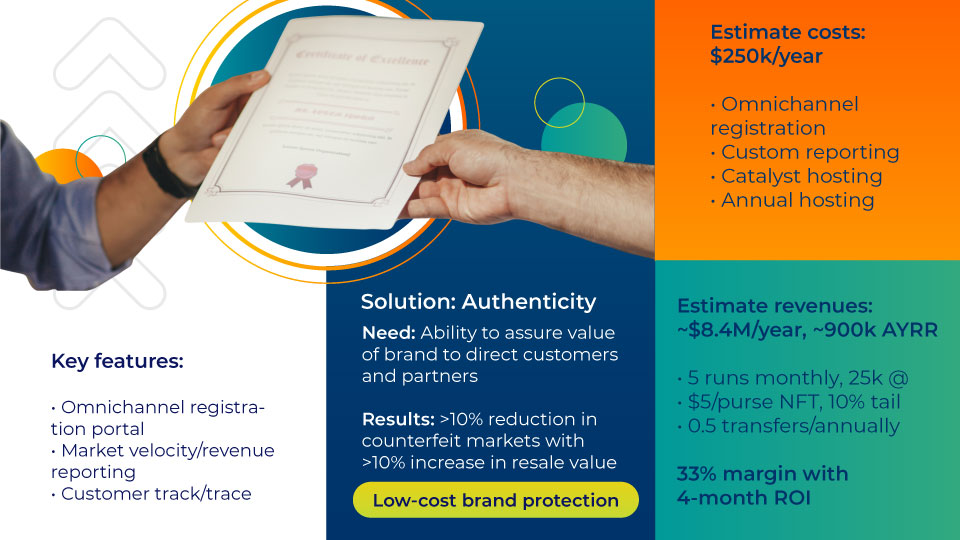
Let’s look at another use case, where you can provide a valuable, 100% digital product, like a media file—audio, visual, or audio-visual. We’ve built a use case around an immersive mobile application like Nike built, but this one is for watches. Consumers get exclusive, limited-edition designs from their favorite designers. The use case for the brand would be testing out new designs—to discover which designs are popular before investing millions of dollars and months to produce them. You would also need an omnichannel marketplace to go with the same portal and reporting solutions from before. The total cost of ownership is $750k with $175k/year. It’s a steep price for a campaign, but a cheap price for a factory to create unlimited digital products for pennies in minutes. The revenue potential here is telling:
- MSRP: $5 with a 10% tail
- 1.5 times a year the watch is re-sold
- Selling the same 1.5M “watch” NFTs a year
This leaves you with an amazing $8.4M revenue stream with a recurring revenue stream of $900k. Yes, this means recurring revenue on products you already manufactured and sold. Our calculations are a 22-day ROI with 91% margin. Eliminating physical manufacturing and distribution shows its upside here.
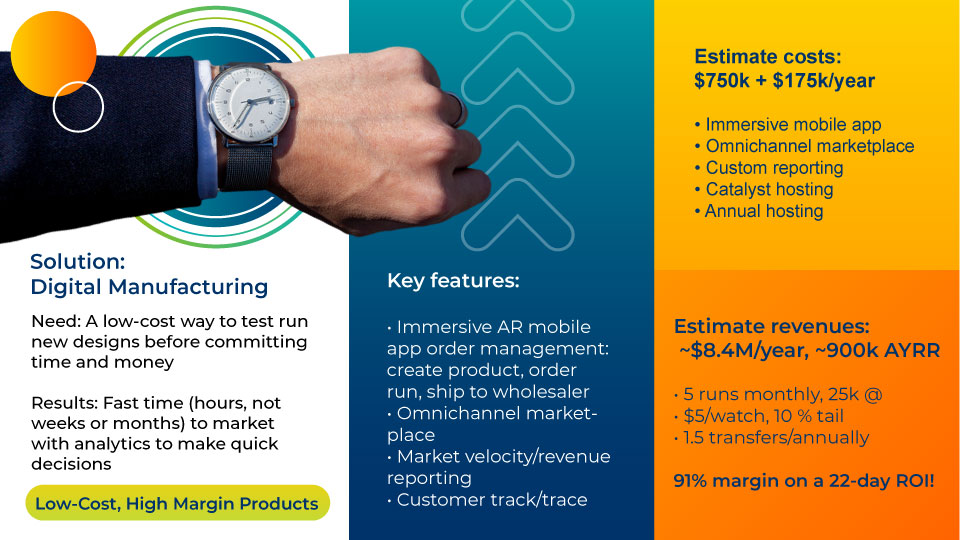
What if you create media content instead of apparel? Imagine seats for an exclusive event or a concert. Web3 can enable secure content digital distribution without using a middleman. The content would use an immersive customer experience, but the delivery method would be the same as before. The key importance of this use case is that you are not tied down by categorization or classification. If you can devise, market, and sell the experience, you can engage the customer using a Web3 lever.
Here are some specifics to the business we use:
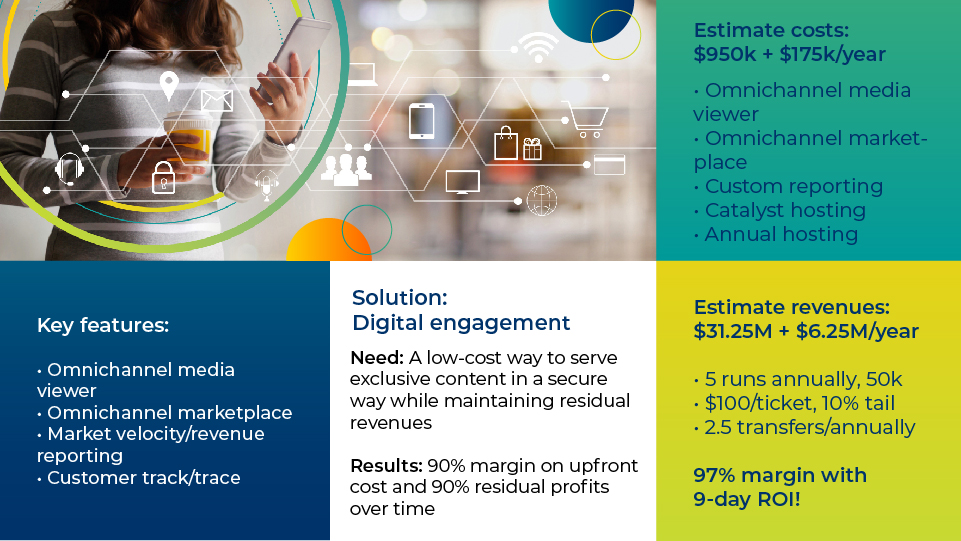
Advocates of Web3 see the ability to reduce latency and intermediaries between the businesses making products and the customers consuming them. They see a fully hosted network connecting us all, free to use to view and track, and one that costs us fractions of pennies for each product produced or sold. Using this technology allows enterprises to connect, serve, and learn from customers directly without the need of physical presence, third-party transaction go-betweens, and/or heavily regulated privacy limitations.
So, what’s the catch? The technology is new and the skills to do this work are limited. You need smart, experienced, and capable people to help you turn Web3 from a pipe dream into real-world products. We offer that expertise. With our turnkey technology, we facilitate test runs of publicly tradeable NFTs at a fixed price. Find out how we can turn your Web3 project into reality.
Why Web3 can improve enterprise CX
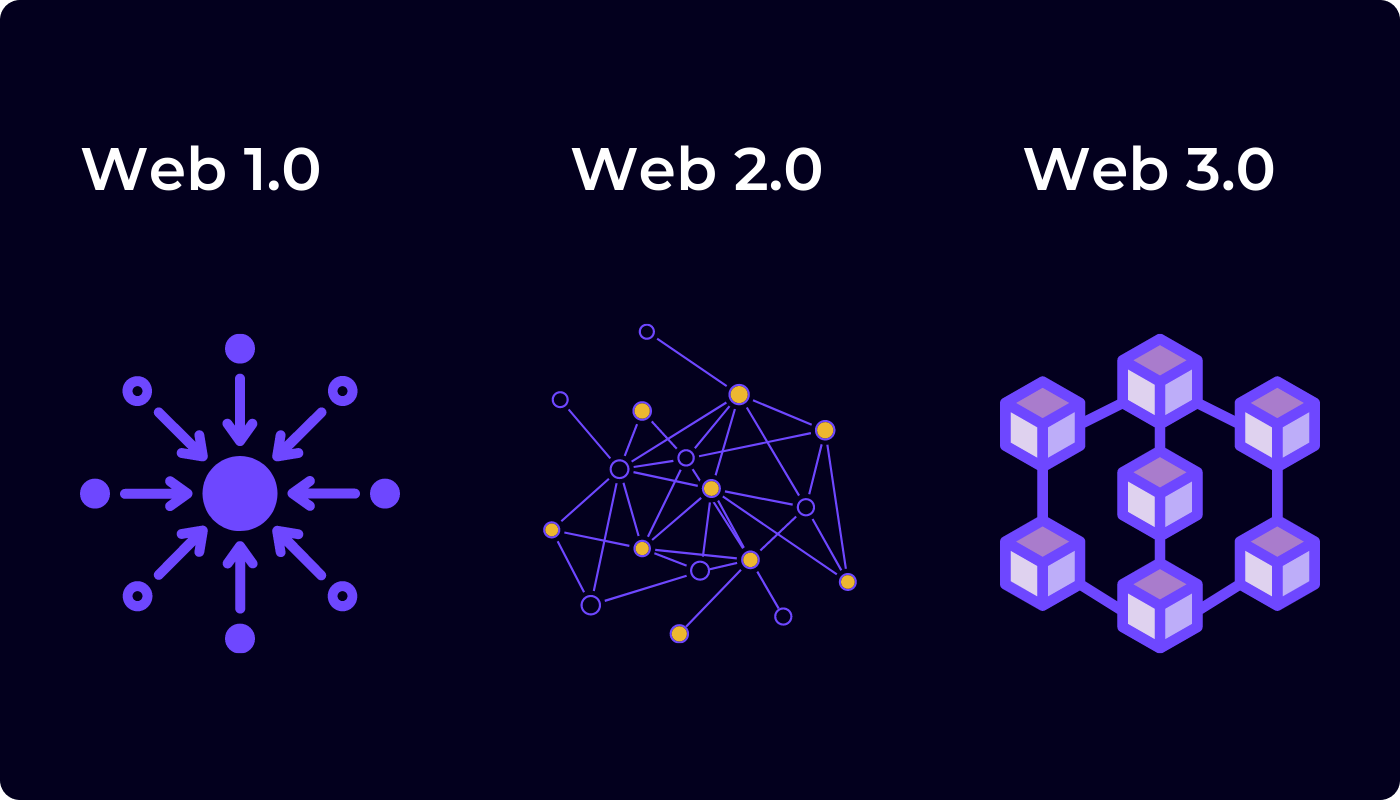
By now, you’ve probably heard the tech buzzword “Web3” in the context of Bitcoin or NFTs of viral memes. Web3, considered to be the next iteration of the internet, is based on blockchain technology, allowing users to read, write, and own their data. However, what you might not know is that Web3 can be leveraged by enterprise organizations to guarantee consumer privacy and security while still allowing for better CX as an outcome.
Here are a few reasons why Web3 can be the future of CX.
Web3 vs. blockchain: A primer
Blockchain is a distributed, decentralized ledger that records transactions securely, permanently, and efficiently. It is not controlled by any one entity, so it has no single point of entry. No one person or entity “owns” the information. The model is highly secure and can be applied to anything of value: currency, personal information, and data of any kind. Think of blockchain as a database arrayed in multiple redundant nodes in many locations. Web3 is the overarching trend that blockchain is a part of —it is the practice of using blockchain to accomplish business solutions beyond simply storing data. Web3 gets its name from its agreed-upon place in the evolution of the internet—the original internet made up of static HTML pages is known as “Web 1.0” and the transition to dynamic social media as Web 2.0. Thus, we’ve arrived at Web 3.0, or Web3.
Blockchain can be leveraged by smart developers to create what are called “smart contracts.” The name is a misnomer, as famous Web3 developers have said that they are neither “smart” nor “contracts.” If you are lost in the minutiae of this terminology, you are not alone. Elon Musk has famously said he is “too dumb to understand smart contracts.”
People get confused thinking that Web3 is the same as cryptocurrency. Though Web3 does include cryptocurrency, the key takeaway of this technology is that it can serve as a building block for enterprise solutions. It’s available to you turnkey and hosted in a public, private, and hybrid way—just like virtualized computers and databases—and adding blockchain to many enterprise solutions generates concrete business value.
But just because a technology is “cool” and hyped does not mean it’s relevant to your business. There is a lot of froth in the world of blockchain, so not all solutions branded as Web3 will yield much, if any, use value. The tenets of Web3, however, do allow for a more decentralized approach to applications and business services.
Why Web3 fits into CX strategy
Companies engage with customers where they are at. Nobody lives in the cloud. From an organizational perspective, however, the traditional challenge of being “everywhere all the time” for customers is expensive—for hosting, managing, and dealing with complexity. One such challenge of needing to be everywhere all the time is enabling point of sale and currency transactions. This is the origin story of blockchain to cryptocurrency to decentralized applications (dApps).
Why does Web3 matter to the enterprise? A turnkey hosted decentralized application framework with the trust and privacy of blockchain built in means fertile ground for B2C applications, by allowing for companies to be everywhere all the time. Web3 makes blockchain technology actionable.
Let’s say we want to create a certificate of authenticity for a physical product we market, manufacture, and sell (like Nike does). Remember the goal: we want to tie our customers to our brand. We need a record of ownership that our customers find value in now and over time. In a Web3 world, that is called an NFT—non-fungible token (a fancy way to say record of ownership). The art and card trading world is in the process of reinventing itself with this technology, but the same technology can be leveraged by enterprises.
This digital artifact can be given to a customer. That customer keeps it in their digital wallet (fancy way to say blockchain user account). When the customer wants to resell that product or prove ownership it is available 24/7/365 at no cost to you (the manufacturer) or them (the owner).
Get up and running with Web3
Advocates of Web3 see the ability to reduce latency and intermediaries between businesses making products and customers consuming them. They see a fully hosted network connecting us all, free to use to view and track, and one that costs us fractions of pennies for each product produced or sold. Leveraging this technology allows enterprises to connect, serve, and learn from customers directly without the need of physical presence, third party transaction go-betweens, and/or heavily regulated privacy limitations—resulting in a faster, more convenient, and more enjoyable experience for customers.
Implementing IoT for Your Sustainability Journey
You can’t manage what you can’t measure, and this is especially true for implementing IoT sustainability initiatives to reduce operational costs and increase efficiency.
Currently, investors and stakeholders are demanding measurable outcomes and economic benefits from ESG initiatives. Environmental benefits, in the form of energy savings, can return both carbon reduction and energy cost reduction. But achieving large savings, and doing so quickly, is hard to do. In addition, not a lot of people are aware of IoT’s role in accelerating sustainability initiatives, and that implementation is actually economically achievable.
In my latest webinar, I moderated a panel including representatives from Optio3 and Carbon Lighthouse. We discussed how businesses can transition toward measurable sustainability quickly and easily using IoT Edge technology.
If you missed the webinar, or would like to experience it again, watch the video below.
https://pkglobal.com/blog/2021/07/iot-sustainability/
New Webinar – Sustainable Sustainability, A Digital Transformation Journey
Want to call out to all my followers that I have a new webinar scheduled on July 20th, 2021. Please click below, join, and share to all your colleagues and friends. More to follow…
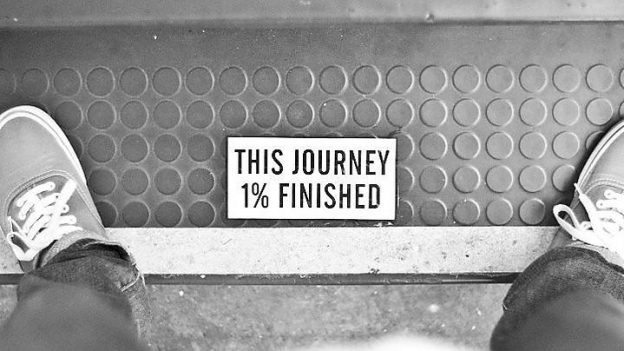
End of the Beginning for Machine Learning
Well thats a wrap for my initial journey into machine learning and artificial intelligence. But it’s only the end of the beginning for machine learning. Before I finish up this series some people have asked that I provide some context. This technology is changing the industry. Some players have already adopted these technologies – my company and competitors. So to placate the masses, let’s list out what I have heard is currently available in the marketplace. I am not omnipotent, so if you see anything missing or wrong please let me know.
Artificial Intelligence is Old Hat

Anomaly Detection vs Chronic Detection
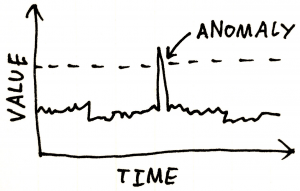
Takeaways
Article Map
- My Journey into Machine Learning with AI
- The Technology of Machine Learning with AI
- Challenges Addressed by Machine Learning with AI
- An Umbrella for Fault Storm Management
- Minimizing Faults with Machine Learning and AI
- Learning Your Operational Performance
- Automated Detection & Mitigation of Chronic Issues
- Service Assurance’s Future with Machine Learning
- End of the Beginning for Machine Learning
Serial entrepreneur and operations subject matter expert who likes to help customers and partners achieve solutions that solve critical problems. Experience in traditional telecom, ITIL enterprise, global manage service providers, and datacenter hosting providers. Expertise in optical DWDM, MPLS networks, MEF Ethernet, COTS applications, custom applications, SDDC virtualized, and SDN/NFV virtualized infrastructure. Based out of Dallas, Texas US area and currently working for one of his founded companies – Monolith Software.
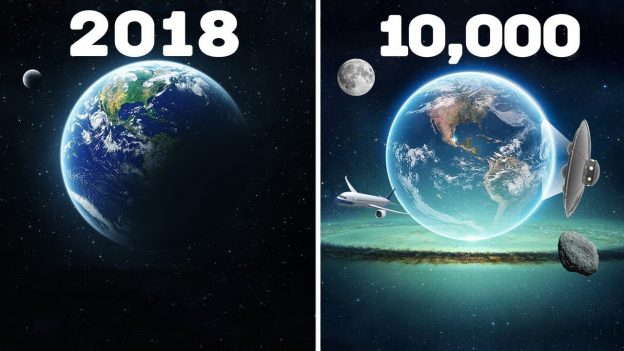
Service Assurance’s Future with Machine Learning
“We Hate Rules” — Says Everyone
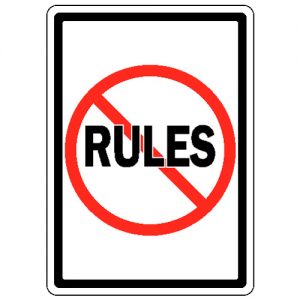
Are We Really Impacting the Customer?

Which KPI is Important?
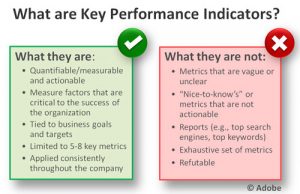
Article Map
- My Journey into Machine Learning with AI
- The Technology of Machine Learning with AI
- Challenges Addressed by Machine Learning with AI
- An Umbrella for Fault Storm Management
- Minimizing Faults with Machine Learning and AI
- Learning Your Operational Performance
- Automated Detection & Mitigation of Chronic Issues
- Service Assurance’s Future with Machine Learning
- End of the Beginning for Machine Learning
Serial entrepreneur and operations subject matter expert who likes to help customers and partners achieve solutions that solve critical problems. Experience in traditional telecom, ITIL enterprise, global manage service providers, and datacenter hosting providers. Expertise in optical DWDM, MPLS networks, MEF Ethernet, COTS applications, custom applications, SDDC virtualized, and SDN/NFV virtualized infrastructure. Based out of Dallas, Texas US area and currently working for one of his founded companies – Monolith Software.
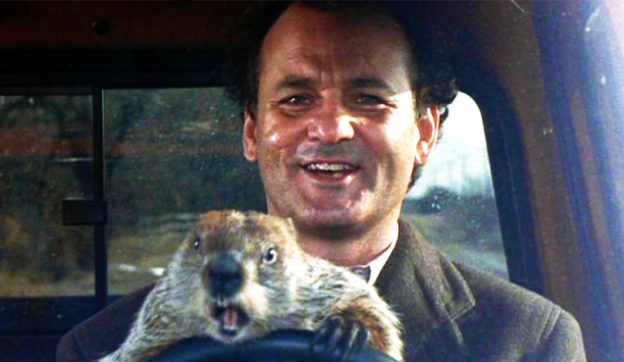
Automated Detection and Mitigation of Chronic Issues
What is an Outage?
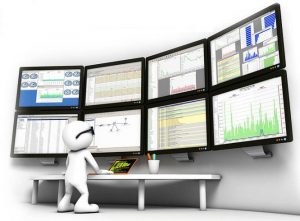
Example – Firmware Bugs
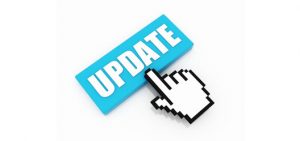
Takeaways
Article Map
- My Journey into Machine Learning with AI
- The Technology of Machine Learning with AI
- Challenges Addressed by Machine Learning with AI
- An Umbrella for Fault Storm Management
- Minimizing Faults with Machine Learning and AI
- Learning Your Operational Performance
- Automated Detection & Mitigation of Chronic Issues
- Service Assurance’s Future with Machine Learning
- End of the Beginning for Machine Learning
Serial entrepreneur and operations subject matter expert who likes to help customers and partners achieve solutions that solve critical problems. Experience in traditional telecom, ITIL enterprise, global manage service providers, and datacenter hosting providers. Expertise in optical DWDM, MPLS networks, MEF Ethernet, COTS applications, custom applications, SDDC virtualized, and SDN/NFV virtualized infrastructure. Based out of Dallas, Texas US area and currently working for one of his founded companies – Monolith Software.

Learning Your Operational Performance
Where Machine Learning Coming Into Play
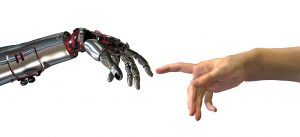
Proactive Workload Management
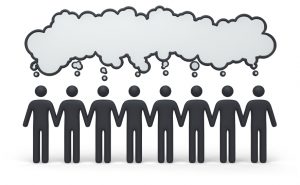
Positive Impact to Operations
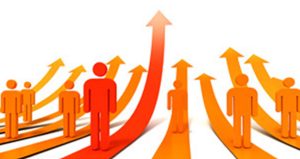
Article Map
- My Journey into Machine Learning with AI
- The Technology of Machine Learning with AI
- Challenges Addressed by Machine Learning with AI
- An Umbrella for Fault Storm Management
- Minimizing Faults with Machine Learning and AI
- Learning Your Operational Performance
- Automated Detection & Mitigation of Chronic Issues
- Service Assurance’s Future with Machine Learning
- End of the Beginning for Machine Learning
Serial entrepreneur and operations subject matter expert who likes to help customers and partners achieve solutions that solve critical problems. Experience in traditional telecom, ITIL enterprise, global manage service providers, and datacenter hosting providers. Expertise in optical DWDM, MPLS networks, MEF Ethernet, COTS applications, custom applications, SDDC virtualized, and SDN/NFV virtualized infrastructure. Based out of Dallas, Texas US area and currently working for one of his founded companies – Monolith Software.